Charles P. Hong1, Tarun Vinodkumar2, Heriberto Andres Nieves-Vazquez1, Jun Ueda2
1Wallace H. Coulter Department of Biomedical Engineering, Georgia Institute of Technology, Atlanta, GA 30332
2George W. Woodruff School of Mechanical Engineering, Georgia Institute of Technology, Atlanta, GA 30332
Abstract:
This paper introduces a sensor-embedded tissue phantom designed to improve Magnetic Resonance Elastography (MRE) testing. By embedding accelerometers within a silicone-based phantom, the study aimed to assess actuator contact quality and wave propagation. A Support Vector Machine (SVM) model was used to classify contact quality with high accuracy (94.53% validation, 90.91% test accuracy). This setup can enhance MRE actuator testing before clinical implementation.
Introduction
Magnetic Resonance Elastography (MRE) is a non-invasive imaging technique used to quantify tissue stiffness. However, actuator contact inconsistencies lead to poor image quality. This research aims to develop a sensor-embedded tissue phantom to test MRE actuators outside clinical environments, reducing mechanical failures and optimizing actuator design.
Methods
Phantom Fabrication
The phantom is composed of SORTA-Clear 37 silicone, embedded with InvenSense MPU6050 accelerometers. These sensors capture shear wave propagation data. The silicone material was chosen for its durability and non-conductive properties. Custom molds were 3D-printed using PLA, ensuring precise sensor placement during the curing process.
Experimental Setup
A modular testbed was designed with adjustable phantom holders and a voice coil actuator (VCS24-029-LB-12). The actuator was controlled via a Galil DMC-30011 controller, enabling precise actuation at varying frequencies. A light displacement sensor (opto-NCDT 2200) was used to verify actuation frequency, interfaced with an Arduino microcontroller for real-time data acquisition.
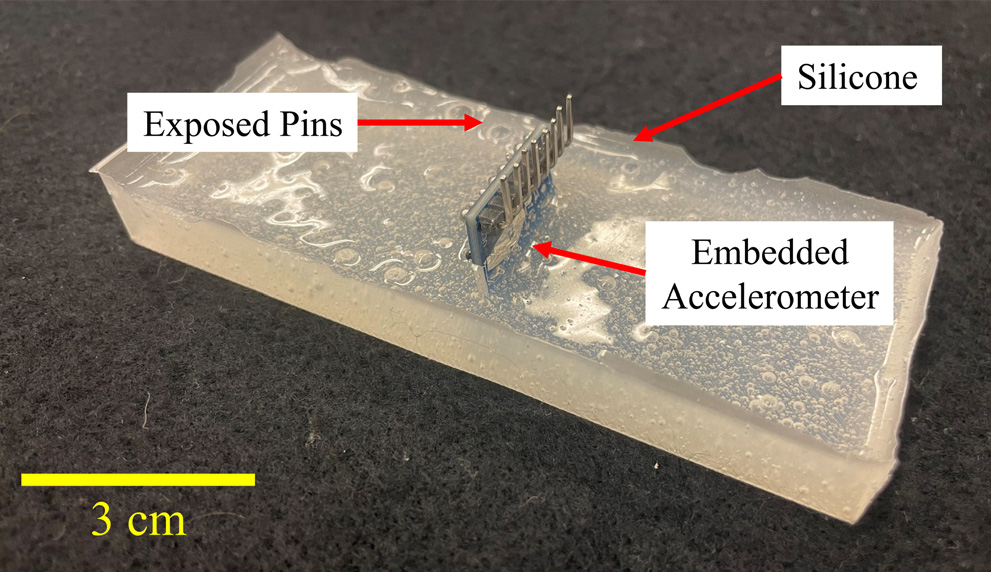
Validation Experiments
- Symmetry Test: Two accelerometers placed symmetrically in the phantom to validate uniform wave propagation. Data from both sensors were cross-correlated, showing an average coefficient of 0.9551.
- Frequency Analysis: A light displacement sensor recorded actuation frequencies (5Hz, 7Hz, and 9Hz) to confirm sensor accuracy, showing perfect correlation (R=1) between the induced and measured frequencies.
- Mechanical Failure Classification: Varying actuator-to-phantom distances (0–840µm) tested contact quality, with 10 trials per interval.
Results
Experiments confirmed that the phantom accurately measures shear wave propagation. The root mean square error (RMSE) between symmetrical sensors was 0.0146, and p-value analysis (p=0.3919) confirmed no significant difference between opposing sensor data. The recorded frequencies precisely matched the actuation source.
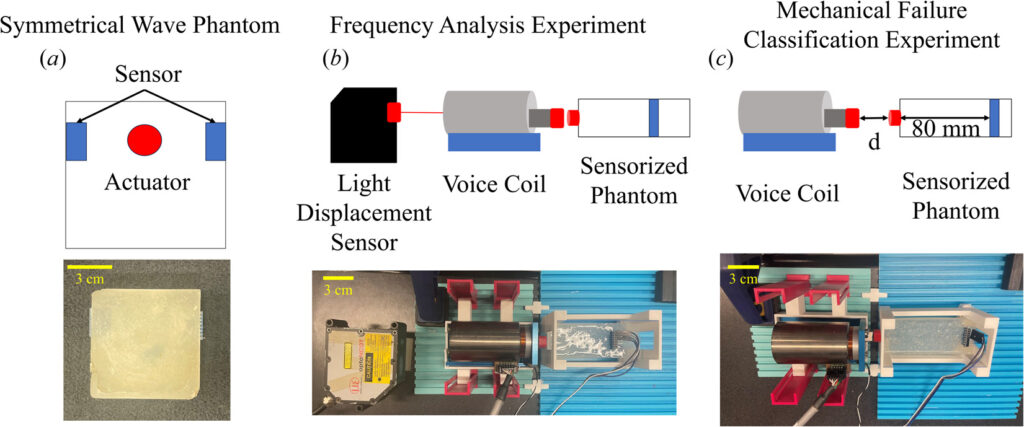
SVM Classification Performance
150 trials were used to train the SVM classifier. Features were extracted from both the time and frequency domains, with feature selection based on correlation analysis. The final model used mean absolute value (MAV), prominence, and peak acceleration for classification. The Fine Gaussian SVM achieved:
- Validation accuracy: 94.53%
- Test accuracy: 90.91%
- Improved performance over linear regression and other SVM models
Discussion
The developed system successfully automates MRE actuator contact evaluation. The embedded sensors reliably capture waveforms, and the SVM classifier provides robust detection of poor actuator contact. The results show a strong negative correlation (R=-0.76) between actuation distance and signal strength in the range of 0-360µm. Beyond 360µm, residual vibrations introduced noise, but the classifier still maintained high performance.
By pre-testing MRE actuators in a controlled laboratory setup, researchers can reduce clinical failure rates, improve actuator positioning techniques, and refine actuator designs. Future work will focus on higher-frequency actuation (40–150Hz) and validation with different MRE actuator types.
Conclusion
The sensor-embedded phantom, combined with machine learning, provides a novel approach to testing MRE actuators outside clinical settings. This method enables quantitative assessment of contact quality, reducing mechanical failure cases and improving the reliability of MRE imaging.
Acknowledgments
The authors acknowledge support from Georgia Tech’s President’s Undergraduate Research Award and the Peer-Reviewed Medical Research Program Discovery Award from the U.S. Army (Award No. W81XWH20-1-0043).
Citation
Hong, C., Vinodkumar, T., Nieves, H., and Ueda, J., “Sensor-Embedded Tissue Phantom For Magnetic Resonance Elastography Mechanical Failure Testing,” ASME Journal of Medical Devices, 2024, MED-24-1046. https://doi.org/10.1115/1.4066226